Key-Locked Rank One Editing for Text-to-Image Personalization - NVIDIA Research
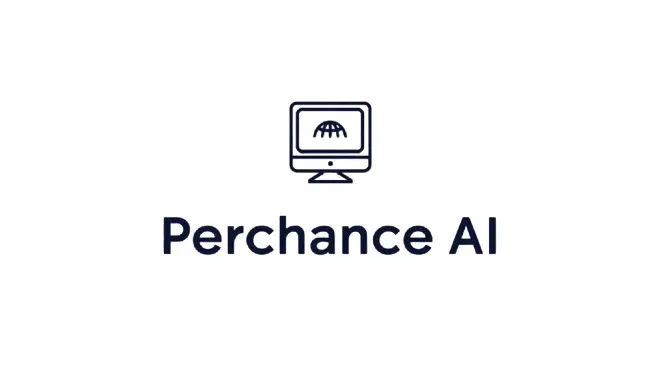
Perfusion enables more animate results, with better prompt-matching and less susceptibility to background traits from the original image. It also allows for efficient control of the visual-textual alignment at inference time.
A mechanism that 'locks' new concepts' cross-attention Keys to their superordinate category, allowing for more visual variability and accurately portraying the nuances of an object or activity.
An approach that controls the influence of a learned concept during inference time and combines multiple concepts.
Perfusion allows for efficient control of the visual-textual alignment at inference time, enabling users to balance visual-fidelity and textual-alignment with a single trained model.
Perfusion can generate images with both high visual-fidelity and textual-alignment when training with a single image.
A Perfusion concept trained using a vanilla diffusion-model can generalize to fine-tuned variants.
Generate images with high visual-fidelity and textual-alignment for personalized advertising.
Create customized product designs with specific features and attributes.
Develop personalized avatars for virtual reality applications.
Generate images for personalized storytelling and content creation.
Train a Perfusion model using a dataset of images and corresponding text prompts.
Use the trained model to generate images with high visual-fidelity and textual-alignment.
Fine-tune the model for specific applications and domains.
Experiment with different hyperparameters and techniques to improve performance.