Video Latent Diffusion Models - High-Resolution Video Synthesis
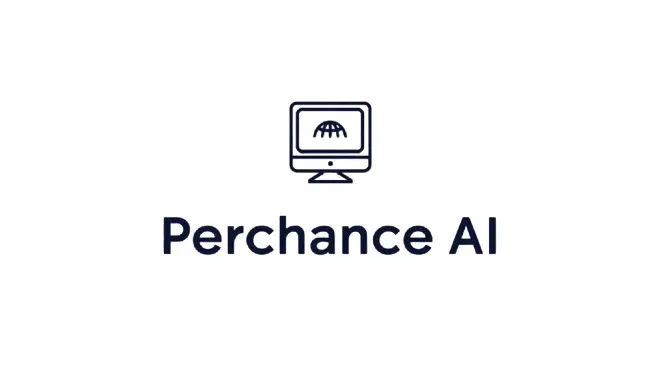
Video LDMs map videos into a compressed latent space and model sequences of latent variables corresponding to the video frames. They initialize the models from image LDMs and insert temporal layers into the LDMs' denoising neural networks to temporally model encoded video frame sequences.
Video LDMs generate temporally coherent videos by modeling sequences of latent variables corresponding to the video frames.
Video LDMs can generate high-resolution videos by leveraging spatial diffusion model upsamplers and temporally aligning them for video upsampling.
Video LDMs can generate personalized videos by inserting the temporal layers that were trained for our Video LDM for text-to-video synthesis into image LDM backbones that we previously fine-tuned on a set of images following DreamBooth.
Video LDMs can generate long videos by applying our learnt temporal layers convolutionally in time.
Video LDMs can simulate in-the-wild driving data by training a bounding box-conditioned image-only LDM and leveraging this model to place bounding boxes to construct a setting of interest.
Generate high-quality videos for creative content creation
Simulate in-the-wild driving data for autonomous vehicle training
Create personalized videos for social media and advertising
Generate long videos for film and television production
Train a Video LDM on a dataset of videos
Fine-tune the model on a specific task or application
Use the model to generate high-quality videos
Experiment with different architectures and hyperparameters to improve performance