UniAnimate: Taming Unified Video Diffusion Models for Consistent Human Image Animation
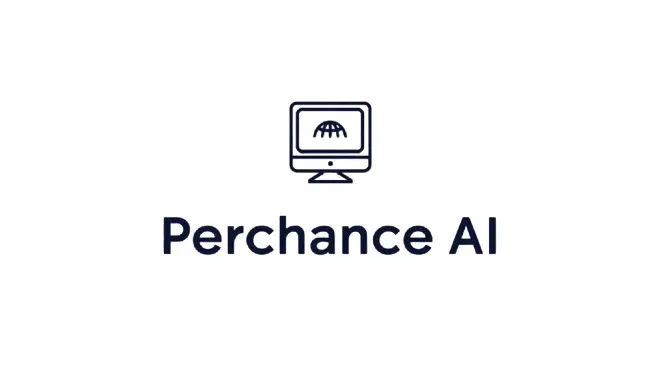
UniAnimate achieves superior synthesis results over existing state-of-the-art counterparts in both quantitative and qualitative evaluations, and can generate highly consistent one-minute videos.
Maps the reference image along with the posture guidance and noise video into a common feature space.
Supports random noised input as well as first frame conditioned input, enhancing the ability to generate long-term video.
An alternative temporal modeling architecture based on state space model to replace the original computation-consuming temporal Transformer.
Generating highly consistent one-minute videos by iteratively employing the first frame conditioning strategy.
Enabling efficient and long-term human video generation.
Improving the quality of video generation and animation.
Utilize the CLIP encoder and VAE encoder to extract latent features of the given reference image.
Employ a pose encoder to encode the target driven pose sequence and concatenate it with the noised input.
Feed the concatenated noised input into the unified video diffusion model to remove noise.