Champ: Controllable and Consistent Human Image Animation with 3D Parametric Guidance
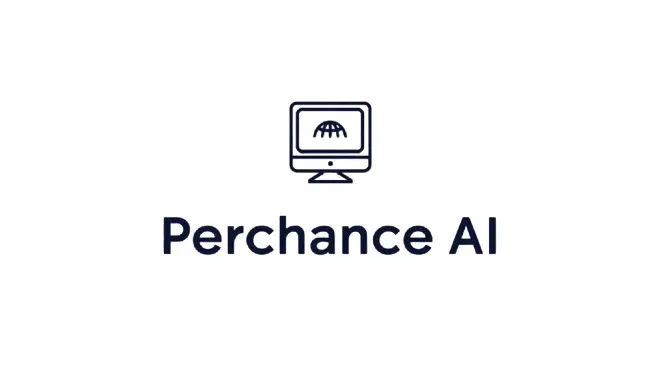
Champ utilizes the SMPL model as the 3D human parametric model, incorporates rendered depth images, normal maps, and semantic maps, and employs a multi-layer motion fusion module to fuse shape and motion latent representations.
Champ uses the SMPL model as the 3D human parametric model to establish a unified representation of body shape and pose.
Champ leverages a latent diffusion framework to enhance shape alignment and motion guidance in current human generative techniques.
Champ employs a multi-layer motion fusion module to fuse shape and motion latent representations in the spatial domain.
Champ integrates self-attention mechanisms to facilitate accurate capture of intricate human geometry and motion characteristics.
Champ performs parametric shape alignment of the human body between the reference image and the source video motion.
Generate high-quality human animations that accurately capture both pose and shape variations.
Create controllable and temporally coherent visual outputs for various applications.
Improve generalization capabilities on wild datasets for real-world applications.
Enhance shape alignment and motion guidance in current human generative techniques.
Input a human image and a reference video depicting a motion sequence.
Use the SMPL model as the 3D human parametric model to establish a unified representation of body shape and pose.
Incorporate rendered depth images, normal maps, and semantic maps obtained from SMPL sequences.
Employ a multi-layer motion fusion module to fuse shape and motion latent representations in the spatial domain.